
AI in Sales Forecasting: The Key to Smarter Predictions
AI in sales forecasting is the fact of using AI to analyze your sales data and predict what might happen in the future. It helps sales teams to make better decisions, be more accurate, and save time by automating a lot of the work.
Core Applications of AI in Sales Forecasting
Automating Routine Forecasting Tasks
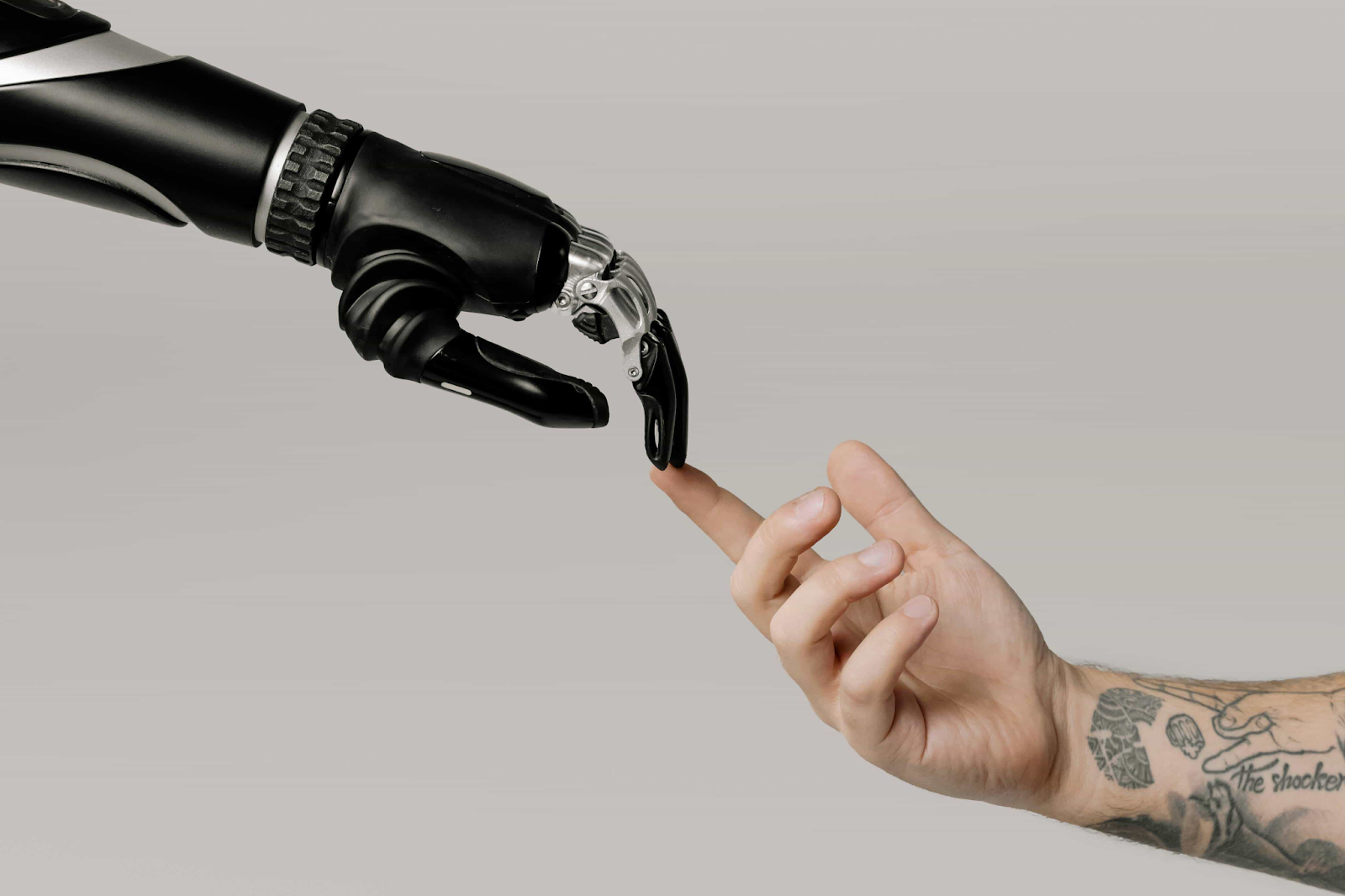
You can use AI-powered sales tools to handle routine tasks easily which will improve the accuracy of your sales forecasting and liberate time for your sales team. Instead of manually entering data or analyzing numbers, you can let AI do it and thus ensure everything is accurate. This way, your team can focus on what they do best : connecting with clients and closing deals.
Furthermore, AI reduces human errors dramatically. You'll get more reliable forecasts with less manual work.
Identifying and Predicting Market Trends
AI is great at going through large amounts of data. They spot markets trends before they become obvious. AI looks at customer behavior, market conditions, and past sales to predict future trends. Companies can now anticipate customer behaviors and market shifts with remarkable precision and keep an advantage over their competitors by responding to these trends quickly.
Enhancing Pipeline Visibility for Sales Teams
With AI tools you will see exactly where each potential customer is in the sales pipeline. Thus, you can quickly identify and remove bottlenecks. These insights can help your teams to prioritize the most promising leads effectively.
Tailoring AI to Industry Needs
Different industries can benefit from AI in their sales forecasting:
- Retailers to predict seasonal demand accurately
- SaaS companies to find customer engagement opportunities
- Manufacturers to optimize resource allocation
- Healthcare to providers manage inventory more intelligently
Popular AI Technologies Powering Sales Forecasting
Machine Learning for Pattern Recognition
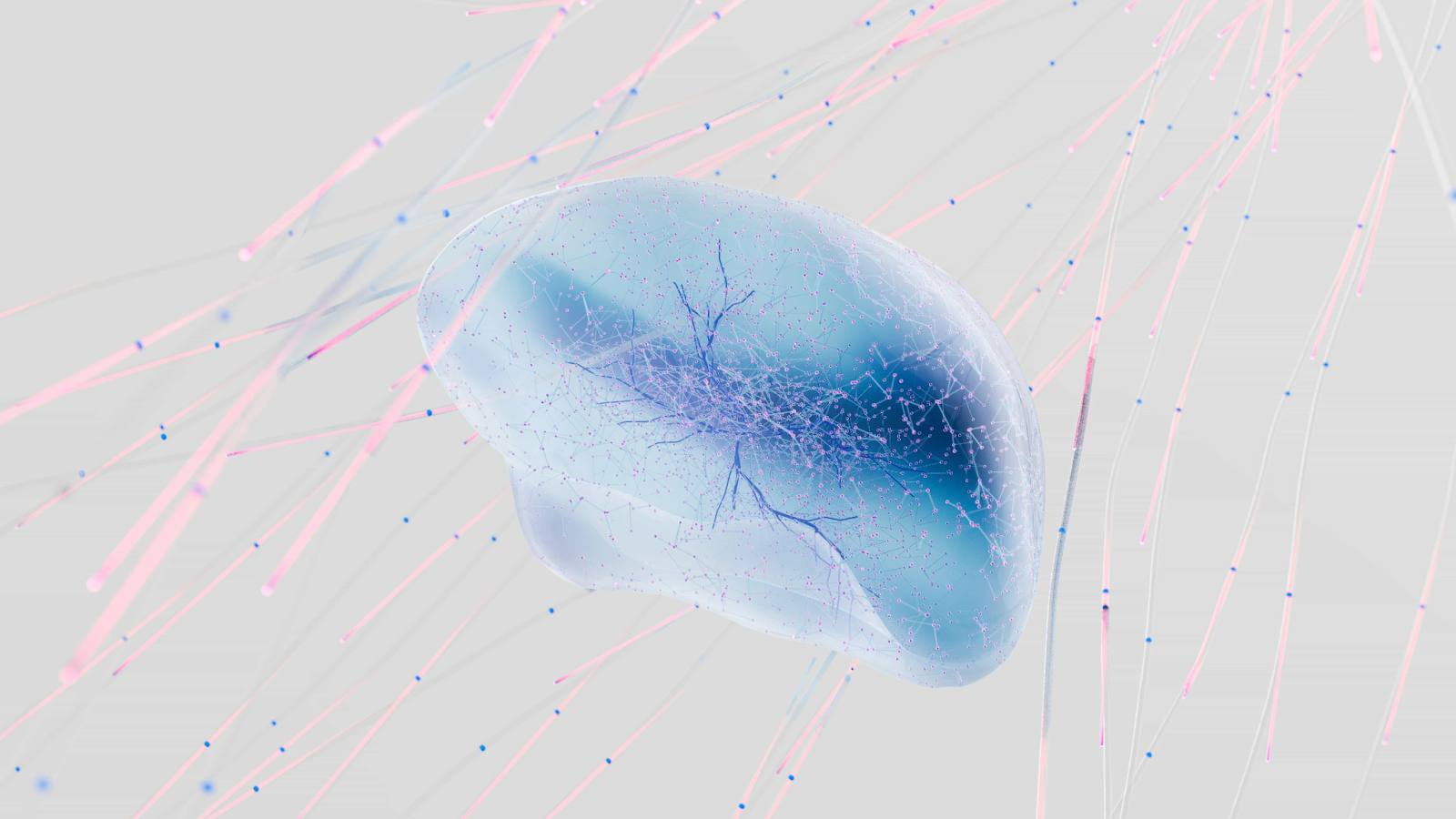
Machine learning is a branch of AI that focuses on enabling systems to learn from data and improve over time without being programmed so. In the context of sales forecasting, these algorithms of machine learning allow to improve predictions over time by analyzing historical data to predict future outcomes. They help sales teams make more informed decisions by recognizing patterns which lead to better performances.
Predictive Analytics and Advanced Modeling
Predictive analytics is the fact of creating complex statistical models to predict future sales scenarios. It considers multiple factors like customer behavior and economic conditions. You can now see potential business outcomes before they happen.
NLP for Understanding Customer Signals
Natural Language Processing (NLP) is a technology that helps machines to understand customer communications deeply by analyzing emails, social media, and support conversations. You’ll be able to understand customer needs. By understanding these signals, sales teams can adjust their strategies and have a better forecasting accuracy.
Business Use Cases: How AI Is Transforming Sales Forecasting
Anticipating Customer Churn
Customer churn refers to the loss of existing customers. By analyzing interaction patterns, AI is able to identify earlier the clients which are likely to leave. This allows your team to take proactive retention steps.
Optimizing Resource Allocation and Team Performance
Resource allocation can be tricky. You can use AI to make smarter resource allocation decisions. AI-powered tools can analyzes performance metrics and market conditions so that your team can focus on the most promising opportunities.
Success Stories: AI Transforming Sales Forecasting
Alibaba's Aliformer: Transforming Sales Forecasting with AI

Alibaba developed an innovative model called Aliformer to predict the sales of millions of products on its e-commerce platform, Tmall. Unlike traditional forecasting methods that rely only on historical data, Aliformer incorporates future knowledge, such as the anticipated impact of upcoming promotions, to enhance forecast accuracy. Deployed on Tmall, Aliformer has significantly improved forecasting performance, leading to better product selection and greater economic benefits.
Danone: Machine Learning for Trade Promotion Forecasting

Danone leveraged machine learning technologies to improve trade promotion forecasting for a range of fresh products with dynamic demand and short shelf life. This initiative achieved a forecast accuracy of 92%, boosting service levels to 98.6%. Additionally, it resulted in a 30% reduction in both lost sales and product obsolescence. These outcomes demonstrate the power of AI in addressing the unique challenges of the food industry.
Claap: Simplifying AI in Sales Forecasting
Claap is an AI meeting assistant that helps sales teams turn meeting conversations into actionable insights. It bridges the gap between discussions and accurate forecasts. No detail gets missed.
Key Features Supporting Sales Forecasting
- Automated Meeting Insights: Claap’s AI captures and summarizes key points and decisions from sales calls. These summaries integrate directly into your CRM, keeping your forecasts accurate without manual effort.
- Collaborative Feedback: With features like video trimming and instant sharing, Claap enables teams to collaborate on meeting insights. This ensures that sales, marketing, and operations stay aligned on key priorities.
Why Claap is Essential for Sales Forecasting
By integrating Claap into their processes, sales teams can:
- Streamline workflows with automated data updates.
- Enhance forecast accuracy by relying on real-time insights.
- Focus on strategy rather than manual tasks.
- Collaborate effectively with clear, actionable meeting data.
In the context of AI in sales forecasting, Claap stands out as it transforms meetings into a source of competitive advantage.
Discover how Claap can help you with your sales forecasting. Try it now with a 14-day free trial and see the impact for yourself.
Challenges to Overcome When Implementing AI
Data Quality and Availability Issues
One of the biggest challenges when implementing AI in sales forecasting is making sure that your data is accurate, complete, and unbiased. Poor data can lead to incorrect forecasts, so you should invest in robust data management before implementing AI sales tools.
Overcoming Resistance to Change
You might face resistance when introducing AI in your sales forecasting, especially if your team is used to traditional methods. To overcome this, introduce AI gradually to your sales team, show how it makes their work easier and provide training and highlight AI’s benefits.
Balancing Human Expertise with AI Predictions
AI is powerful, but human expertise is still a big part of sales forecasting. Sales professionals need to interpret AI-generated forecasts and use their experience to make the best decisions. The sales forecasts come from combining AI with human intuition.
Ethics in AI Forecasting
The use of AI in sales forecasting raises ethical concerns, particularly around the use of customer data. It is important to ensure compliance with regulations like GDPR, which protect individual privacy and limit how companies can use personal data. Companies must be transparent about how they use data, obtain explicit consent, and ensure that their practices respect customers rights.